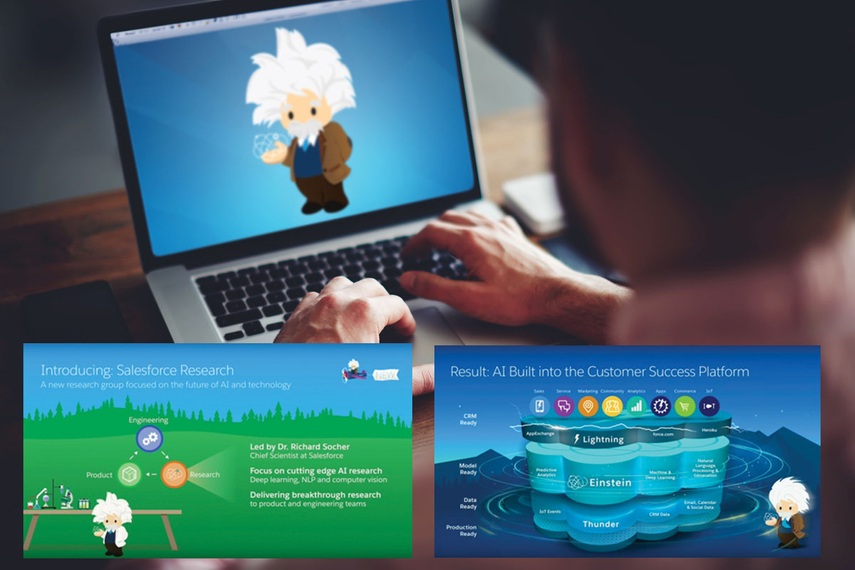
The pitch was certainly compelling enough. Speaking on the side-lines of Dreamforce, Salesforce’s annual conference in San Francisco, Derek Laney, head of product marketing for Salesforce in Asia was doing his part to reinforce the message from the event’s biggest product announcement — Einstein.
The company’s latest offering will embed artificial intelligence (AI) in the Salesforce Platform. This AI layer is touted as the new tool that will enable marketers to get closer to their customers, automatically discovering relevant insights, predicting future behaviour, proactively recommending best next actions and even automating tasks.
According to Laney, everyone is trying to crack the data-intelligence puzzle. While companies have access to data, many are still seeking a way to make it useful.
“The problem is that the skills required to manage all this data and analyse it, are hard to come by,” he says. “Data scientists are being poached straight out of university and every company in the world is on the hunt for talent.”
Anyone who isn’t the top five or six companies in the world would struggling to create their own internal practices. There’s a supply issue and that leaves mid-market companies with no chance. With the Einstein layer, Salesforce intends to create a generalised AI platform that people can use with minimal technical knowledge.
“This will enable marketers to get more out of their dollar via the insights gleaned from machine learning,” says Laney. “We’re addressing problems that were solvable in the past but required a huge amount of time and resources.”
And Salesforce is far from the only player getting in on this game. In early November, Adobe unveiled Adobe Sensei, a new framework and set of intelligent services for dramatically improving the process of designing, and delivering, digital experiences.
The new offering’s intelligent services promises to tackle complex experience challenges, including image matching across millions of images, understanding the meaning and sentiment of documents, and finely targeting important audience segments.
Just like Einstein, Sensei is built into the company’s cloud offerings, which include Adobe Creative Cloud, Adobe Marketing Cloud and Adobe Document Cloud.
Artificial intelligence as a service?
The consolidation of talent to work on data-driven solutions to common problems for marketers, made accessible to existing customers of marketing software as a value-added service, seems to make economic sense.
David Sanderson, CEO of Nugit, says the importance of data analytics is widely acknowledged but not many marketers are taking genuine steps to embrace the new technologies that have emerged with this important trend.
Jargon guide In its most widely understood definition, AI involves the ability of machines to emulate human thinking, reasoning and decision-making. However, there is some confusion in the marketplace around AI technology and the terminology used to describe it, with many similar-sounding terms often being used interchangeably. Here’s a quick rundown of the most common, and their definitions: Augmented intelligence: The effective use of information technology and systems that augment or enhance human capabilities. Cognitive computing: The simulation of human thought processes in a computerised model. It involves self-learning systems that use data-mining, pattern recognition and natural language processing to mimic the way the human brain works. Machine learning: A type of AI that provides computers with the ability to learn without being explicitly programmed. It focuses on the development of computer programs that can teach themselves to grow and change when exposed to new data. Deep learning: A branch of machine learning concerned with algorithms inspired by the structure and function of the brain, called artificial neural networks. Machine intelligence: Used interchangeably with AI, also refers to systems that are able to learn the structure of streaming data, make predictions and detect anomalies. They also learn continuously from unlabelled data. |
As an AI and data analytics startup, Nugit sees itself as a smaller but more agile player in contrast to the global heavyweights in the space.
Sanderson shares that in client meetings, the refrain “this is how we’ve always done things” is often heard. Clients are concerned with the level of difficulty in learning how to use new tools, as well as the costs and time involved in implementation and training.
“These are very real concerns that should be addressed, but at the heart of it lies the fact — as with anything relatively new — that people are often resistant to change,” he says. “Many of these tools are developed for data analysts with coding skills and not necessarily for marketers who do not have in-depth analytical skills.”
As the shortage in skilled data analysts continues to grow along with the importance of timely insights, more platforms are emerging to help marketers get to the insights they need faster.
“Ease of use and simple visual representations are key to help non-technical marketers adopt these platforms,” he notes.
If you build it, they will come
In terms of adoption of AI systems, an IDC report on Cognitive/Artificial Intelligence Systems Spending Guide showed that the largest spender on such technologies is Japan, followed by Asia-Pacific as a region.
Meanwhile, a Gartner’s CMO Spend Survey 2015-2016, reported that marketing technology represents 33 percent of the marketing budget and from that budget, marketing and analytics software purchased as a service accounted for 24.4 percent.
Forrester expects investment in AI and cognitive capabilities to rise sharply as firms look to tap into complex systems, advanced analytics, and machine learning technology.
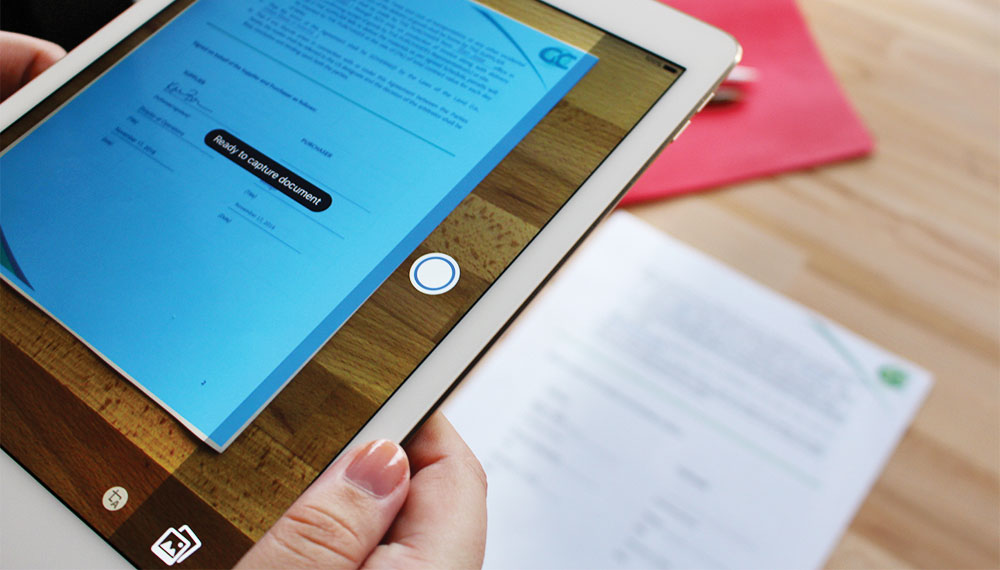
In addition to Salesforce and Adobe, other vendors embedding bits of AI capabilities into their solutions and positioning themselves to prosper include: Google, IBM, Persado, and Squirro.
The race to incorporate AI tools is a response to businesses in need of assistance to cope with an increasingly complicated marketing environment. Michael Barnes, vice-president and research director at Forrester, says marketers must now manage the entire customer life-cycle, running from initial discovery through to purchase and on-going engagement.
“This engagement will be via existing channels as well as new and/or rapidly evolving channels like mobile, wearables and IoT, all driving an on-going data explosion,” he adds. “Applying analytics across the life cycle can help marketing professionals identify opportunities to extend customers’ lifetime value and prompt value-generating behaviours.”
With consumer value the underlying goal, firms acquire more profitable customers through better targeting; reduce the cost of acquisition in the early stages of the relationship; cross-sell and upsell to the right customers; and increase wallet share through loyalty, retention, and recovery programmes.
However Barnes notes that the data explosion of the past few years means more complex data integration and insight generation challenges and requires an analytics capability that is fast, agile, and scalable.
“Moreover, customer analytics now must deliver contextual insights, not just binary, one-dimensional insight about purchase behaviours and marketing response,” he says.
The demands on what analytics tools must deliver are high, and it’s easy to see how the seeming “magic” that can be accomplished by machine learning algorithms could be seen as some silver bullet for marketing headaches.
But Sanderson cautions against such thinking, adding that it is much more important to have the right data and find quality amidst the quantity.
“Treating it as a silver bullet or as a replacement to real thinking is not the right approach,” he says, adding that such efforts need to support and be in line with a viable business strategy, otherwise the output will not be useful.
“Machines, at least for now, are optimisers and pattern recognisers — you feed them an objective and a problem and they’ll find you an optimal solution. If you can’t define the problem or the objective, then there’s no point because the machine will not find it for you,” he says.
Sanderson says the first consideration should be which tool best solves a marketer’s specific needs — the industry favourite isn’t always the best solution for everyone.
“What answers do you need from your data and where do you need to get it from?” he says. “Ease of use and the level of regional support will also be key in training and on-boarding your teams to embrace a new tool.”