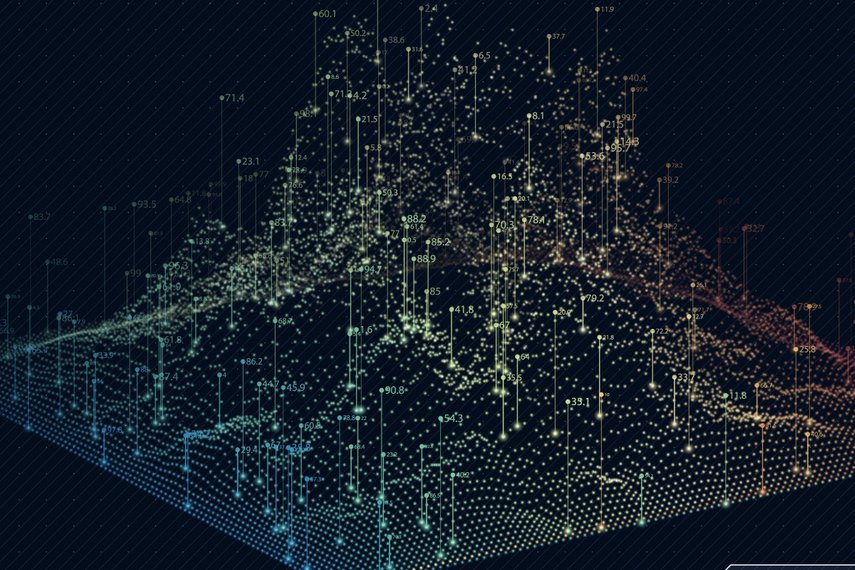
The advertising industry has lurched forward dramatically over the last 10 years, driven by the rapid expansion of digital, which is now beginning to colonise the OOH, radio and TV environments, promising yet more complexity to come.
Ten years ago, measuring your marketing was far simpler, and you could rely on tools like econometrics to create implicit links between advertising and sales and act accordingly.
However, with the recent explosion of digital marketing driving a data revolution, this promised great things for measurement. For the first time an advertiser should be able to start to see the direct relationship between an event and a sale.
The only problem is that despite the promise of big data, the revolution has yet to arrive. This was confirmed in our 2017 study where we found that 66% of advertisers find measurement very challenging.
The messy reality of new technology
Reading the industry press, we are constantly just one step away from realising the promise of this data, with one new piece of technology. Buzzwords abound, machine learning is a great example. However, marketers hoping to invest in these hyped up, expensive technologies, believing they are a cure-all for their problems, will ultimately be disappointed.
Instead, marketers should question why there is a disconnect between opportunity and reality. And why is the great leap forward in these digital technologies is always tomorrow, and never today?
Machine learning, simple, clear, wrong
Take self-driving cars, which rely on extensive machine learning, backed up by human involvement in real time. Small errors in the assumptions made by the designers can lead to disastrous consequences if, for example, localised variants of traffic signals are not included in the dataset.
On the other hand, handled correctly, AlphaGo demonstrated the potential for machine learning, not just performing ahead of almost any human player in the game of Go but also undertaking moves “that would never happen in a human-to-human Go match” according to one opponent Ke Jie. Even in success, this helps demonstrate that a machine learning based system can behave very differently to the human intent.
Applying machine learning to measurement
Attribution, the ability to link preceding events to a sale or some realisation of value, is deceptively simple but it relies on having four things:
- An ability to take in raw data of all types, often from disparate sources and hold them for a sufficient period
- A toolkit suitable to extract that data and perform manipulation on it
- Individuals with the skills to perform the analysis
- A hypothesis to test
Much has been made of machine learning’s ability to crack attribution, but when evaluating why clients have not made progress it is far more common that the challenges are around collecting and handling the data itself, or a lack of access to the relevant skill sets needed to conduct the analysis.
Despite its promise to deliver without human intervention, machine learning often still requires a great deal of human help.
Achieving effective measurement is a marathon, not a sprint
Advertisers need help in solving these complex challenges, but as an industry we need to be comfortable that we are still rebuilding for the digital age.
We would always advise that advertisers take stock, figure out first what they want to achieve, and more importantly why they want to achieve it. Steady measured steps are far more valuable (and likely) than revolutionary change.
Moving between a last touch attribution model to a more complex fractional attribution model will totally change how you value activity. Neither model is wrong, both are right in different ways, and advertisers need to be comfortable with a shifting landscape.
There are no cheat codes, no way to get to the finish line without going the full marathon length.
Technologies like machine learning appear transformative but are still in their infancy. There is a whole toolkit of mature and powerful technologies, along with highly skilled analysts, that are available to help solve advertiser’s challenges today.
Chris Cox is client analytics lead at Infectious Media.