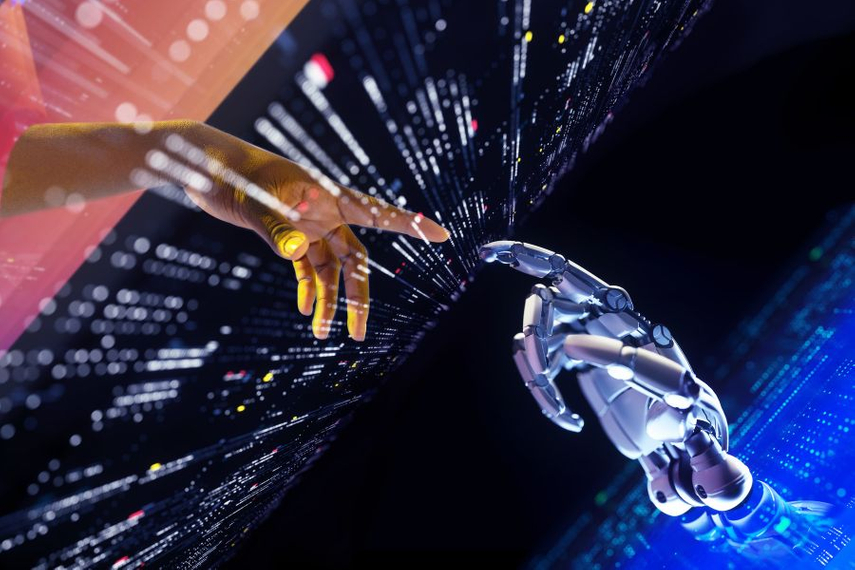
Please sign in or register
Existing users sign in here
Having trouble signing in?
Contact Customer Support at
[email protected]
or call+852 3175 1913
Synthetic data offers a compelling solution to the challenges of data collection and analysis. But can AI-generated C-suite insights truly transform how companies make crucial decisions? B2B International's Amanda Chew explores.
Contact Customer Support at
[email protected]
or call+852 3175 1913
Top news, insights and analysis every weekday
Sign up for Campaign Bulletins
Geekie’s pivotal role in evolving Jaywing’s service offering now brings the agency more than half of its revenue from The Studio, underpinning the importance of performance-driven solutions to marketers.
The Federal Trade Commission’s long-running case against Meta has entered a new legal phase, with Instagram and WhatsApp at the centre of allegations that the tech giant built a social-media monopoly through acquisitions.
Brands are increasingly exploring the lucrative potential of advertising in space, with companies like StartRocket pursuing orbital billboards while others opt for more environmentally friendly near-space marketing alternatives.
Tim Devine moves as AUNZ ECD to global chief invention officer. Plus, new global leads for strategy, technology, and transformation.