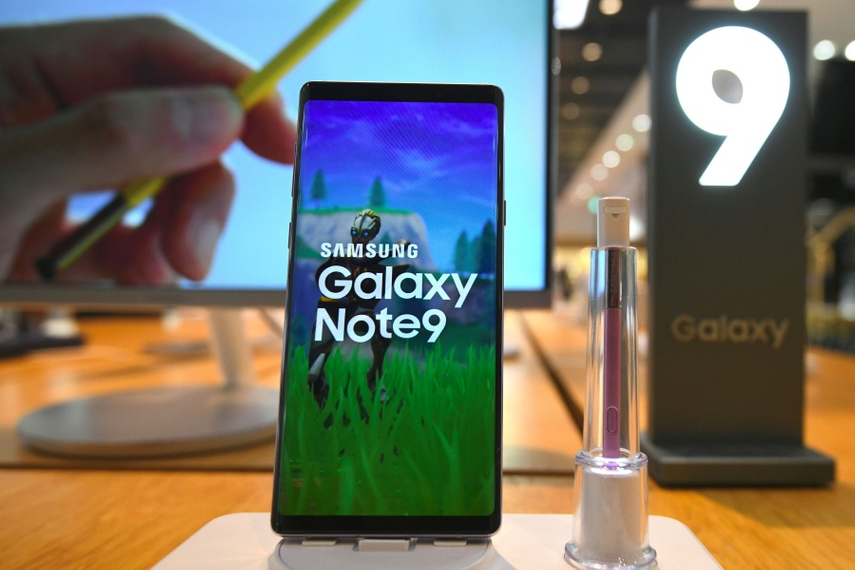
Background and objectives
One of the biggest challenges for marketers today is driving conversions consistently and cost effectively. Working with Verizon Media and Carat (the media agency for StarHub), StarHub used artificial intelligence and programmatic delivery to build a solution to try to address these challenges.
StarHub wanted to target users interested in mobile phones and the latest models—the ultimate objective being to drive purchases of the new Samsung Galaxy Note 9 online. This would be measured by the number of customers landing on the ‘thank you’ page post-order.
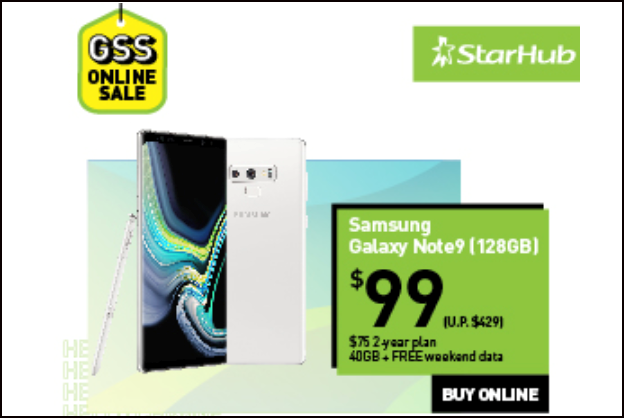
Execution
The strategy was to execute a pure-play DSP (demand-side platform) campaign that relied on Verizon Media’s data, scale and machine-learning capabilities. Verizon Media’s DSP is powered by AdLearn optimisation, which combines demand and supply data with predictive performance algorithms to connect the best ad with the right user and placement, at scale.
The company's Predictive Audiences uses deep learning to devise predictors that can accurately locate potential buyers, thereby mapping, targeting and converting the right audiences from millions of users.
By combining key algorithms from AdLearn with Predictive Audience's ability to predict the purchase probability of each user, Verizon Media created several new consumer segments to target. This combination of AdLearn and Predictive Audience could analyse StarHub’s existing converted users to find the most accurate predictors of purchase.
Users were placed into eight segments, ranging from tier 1, which had a very high probability of conversion but was a very narrow audience, to tier 8, where the probability of conversion was lower but the base was much broader. Verizon Media’s machine-learning based technology then decided on the best mix to serve the campaign.
The campaign ran for two months, from May 4 to July 5 of 2019, with the creative linked to the Great Singapore Sale (GSS) and promoting significant discounts for the Samsung Galaxy Note 9.
Results
The targeting campaign delivered a cost per acquisition (CPA) that was eight times lower than that delivered by lookalike targeting, showing that a predictive model offers increased performance and cost effeciency compared with a model based largely on past behaviours. The conversion results from the audiences targeted by machine-based learning were 2.5 times higher than RON (run of network) advertising.
These findings backed Verizon Media’s internal research, which found that machine learning could help deliver a 48% uplift in conversions, a 38% reduction in cost per click (CPC) and a four to eight-fold reduction in CPA.